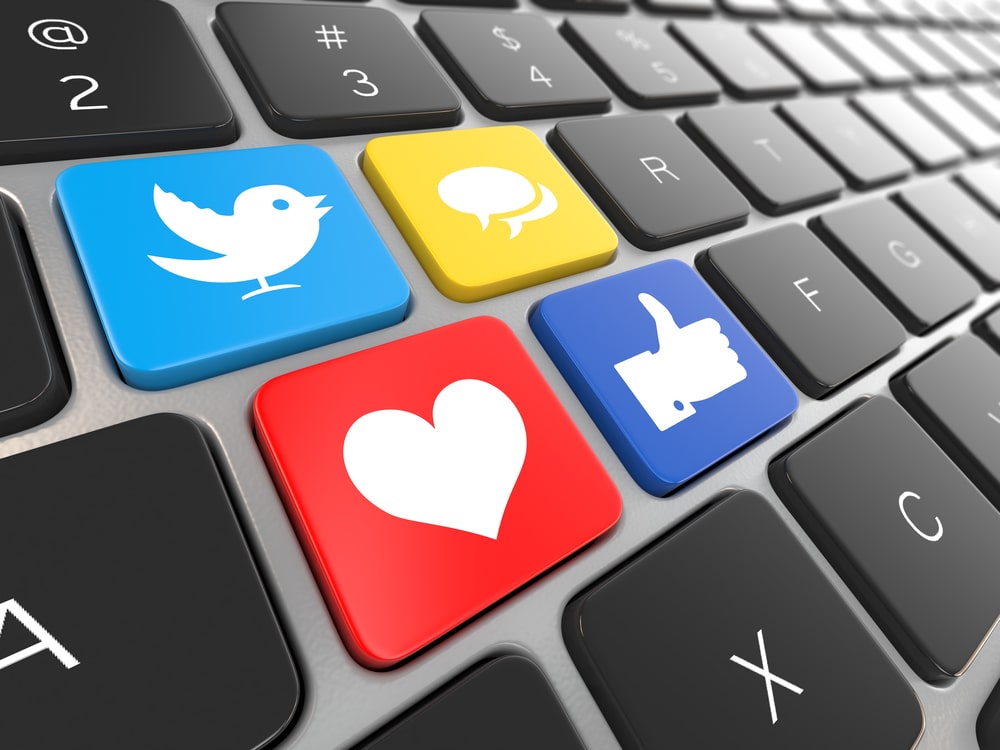
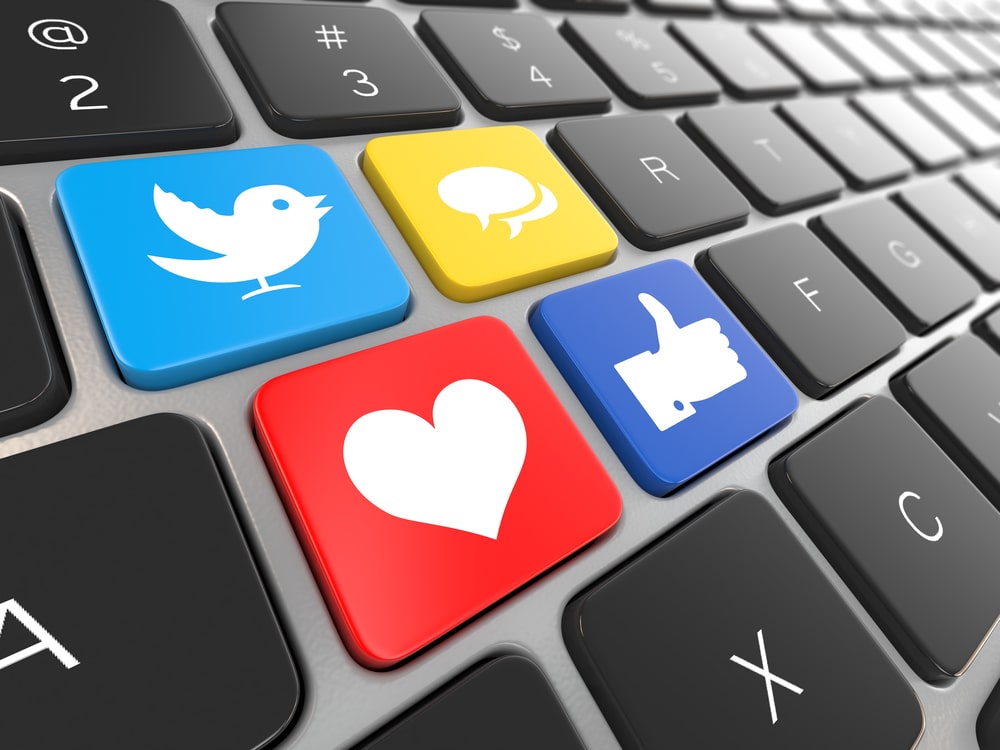
Summary
- A popular Swiss Financial Institute research paper, co-authored by UC Berkeley researchers, reveals the importance of following the right Twitter accounts.
- It finds that only a quarter of ‘finfluencers’ generate positive abnormal returns for their followers. Worryingly, over half generate losses.
- Clearly, picking winners from losers is not easy for the Twitter crowd, as most follow finfluencers with poor track records.
- Typically, the worst finfluencers tweet more often, are overly optimistic, and ride return momentum. In contrast, skilled finfluencers are return-, sentiment-, and news-contrarian.
Introduction
Financial influencers, or ‘finfluencers’, can have considerable sway over the investment decisions of retail investors. Yet, few are registered as investment advisers or brokers. Little is known about the quality of this unsolicited financial advice, and its impact on their follower base, trading activity, and asset prices. Until now.
A new Swiss Financial Instituteresearch paper, downloaded nearly 1,000 times, reveals the low average accuracy and high dispersion in the quality of advice by finfluencers. They find that most financial advisers on Twitter provide advice that generates negative monthly returns and, despite this, they typically have the most followers. Beware!
Data
The authors use tweet data from Bloomberg, influencer data from StockTwits, stock returns from CRSP, and factor returns from Ken French’s website. Their sample period extends from July 2013 to January 2017.
Tweet data from Bloomberg contains the time, content, stock ticker, and the usernames of tweets. It also supplies a social sentiment score based on a proprietary machine learning algorithm. Out of 72mn tweets, 11%/77%/12% are positive/neutral/negative.
StockTwits contains data for each Twitter user, from the number of tweets they have made to the number of followers they have. It also has information on the number of stocks they watch, the number of investment ideas they have generated and the number of likes their tweets have received from other users.
The authors need to match; (i) user data from StockTwits with tweet data from Bloomberg, and (ii) tweets data from Bloomberg with returns data from CRSP. Together, they can collect tweet sentiments by any user for any stock on any day. In total, the paper’s sample includes information on 29,000 finfluencers.
Alpha Generation
Armed with enough detail to determine what trade ideas by which finfluencer yield the highest returns relative to market factors, the authors calculate the monthly estimated alpha generated by each.
They find that the average finfluencer has a monthly estimated alpha of -0.63%, or -7.56% per year. The median is also negative, meaning most users post systematically anti-informative tweets! However, the top 25% generate abnormal returns of 1.86% per month, which is economically large (Chart 1).
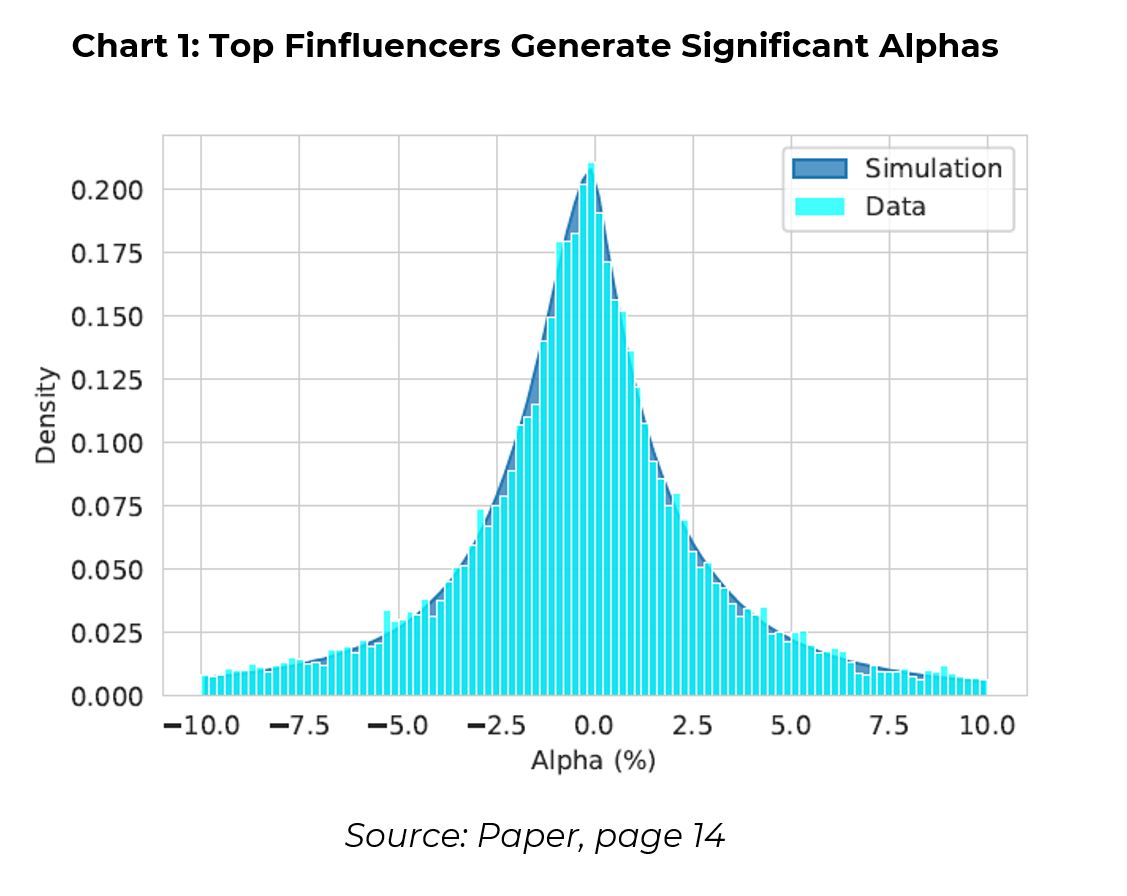
Measuring Finfluencer Skill
Skills can be proxied by the abnormal returns each user generates. The authors use the following classification of finfluencers skills: those generating positive alphas are categorised as ‘skilled’, those with zero alpha-generation are ‘unskilled’, while negative alphas are produced by users categorised as ‘anti-skilled’.
Based on the authors’ calculations, 27.5% of finfluencers are skilled, 16% are unskilled, and 56.5% are anti-skilled. They run simulations over this distribution of skill and produce the probabilities that users are skilled, unskilled, and anti-skilled (Chart 2).
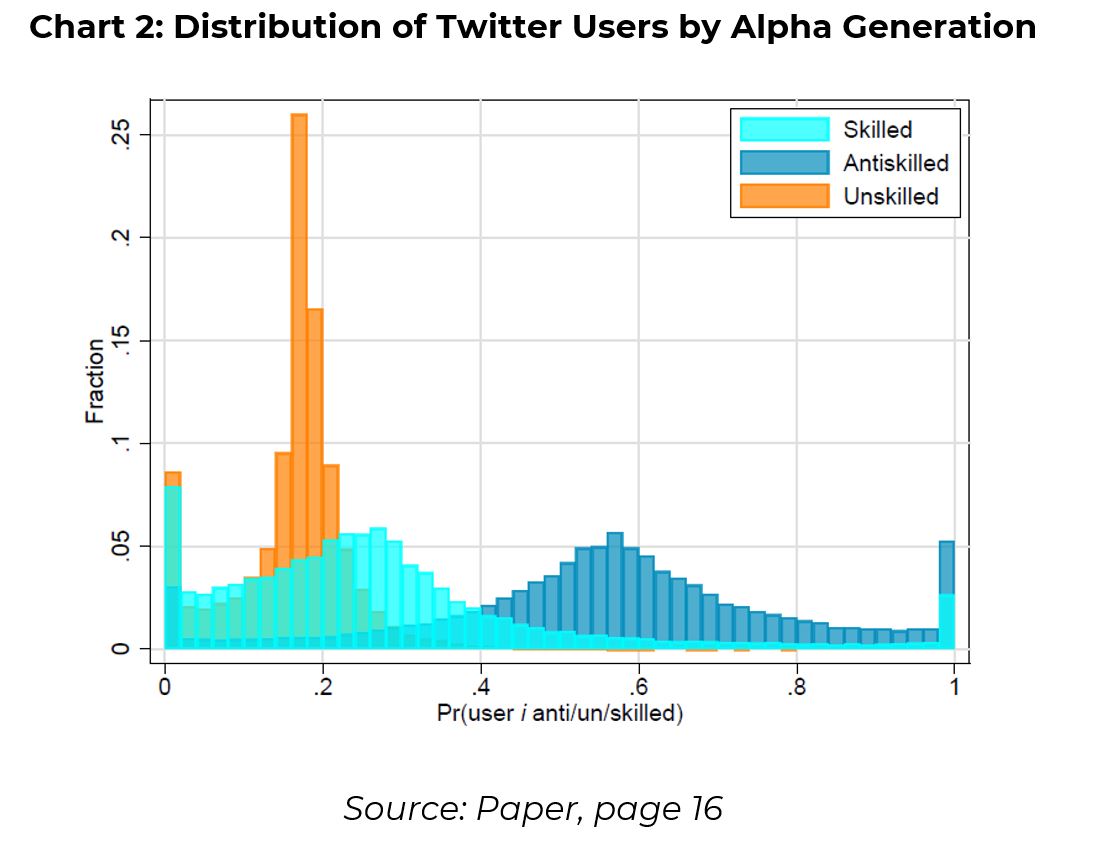
The chart shows that most Twitter influencers have an over 50% probability of being anti-skilled, with almost 5% delivering unambiguously negative returns. If most StockTwits users are anti-skilled, then their tweets contain important information in the sense of ‘do opposite to what I say’!
That only 28% of StockTwits are skilled contrasts with other work showing that 56% of Seeking Alpha users predict stock returns correctly. According to the authors, this reveals that platforms with more curated users have more informative content.
Twitter Users Following the Wrong Influencers
Do more skilled finfluencers have larger followings? No. The authors find a strong negative correlation between the number of followers and the probability of being skilled (Chart 3). In other words, the more skilled an influencer is, the fewer the followers they have.
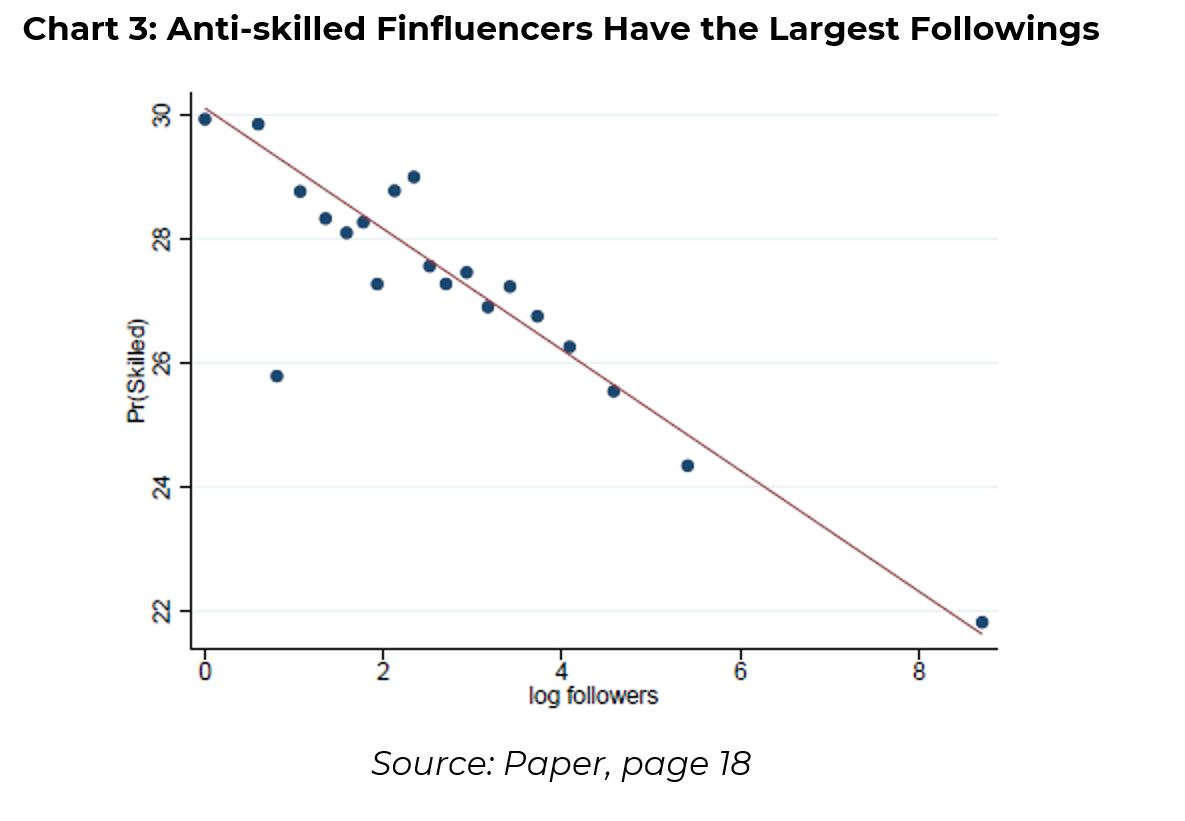
This negative relationship cannot be explained by how long skilled vs anti-skilled finfluencers have been on the platform. Skilled and unskilled influencers are equally as likely to remain on StockTwits throughout the sample and skill does not determine whether an influencer survives as an influencer.
Anti-skilled influencers tend to be market ‘gurus’. They are influential enough to move aggregate retail order balances on the next trading day. Skilled influencers do not have this sway.
There are, however, some predictable relationships between the skill of finfluencers and their followers. Tweets from anti-skilled users strongly predict. For example, positive sentiment tweets lead to higher retail buys over sales. Meanwhile, skilled influencers have no such impact on the aggregate.
The Imposter Strategy
Anti-skilled finfluencers are particularly good at generating tweet activity. Perhaps this is why they feature so heavily on social media platforms. The authors again find a strong negative correlation between skill and the number of times a finfluencer tweets. In other words, the more a finfluencers tweets, the less likely they are to be skilled (Chart 4).
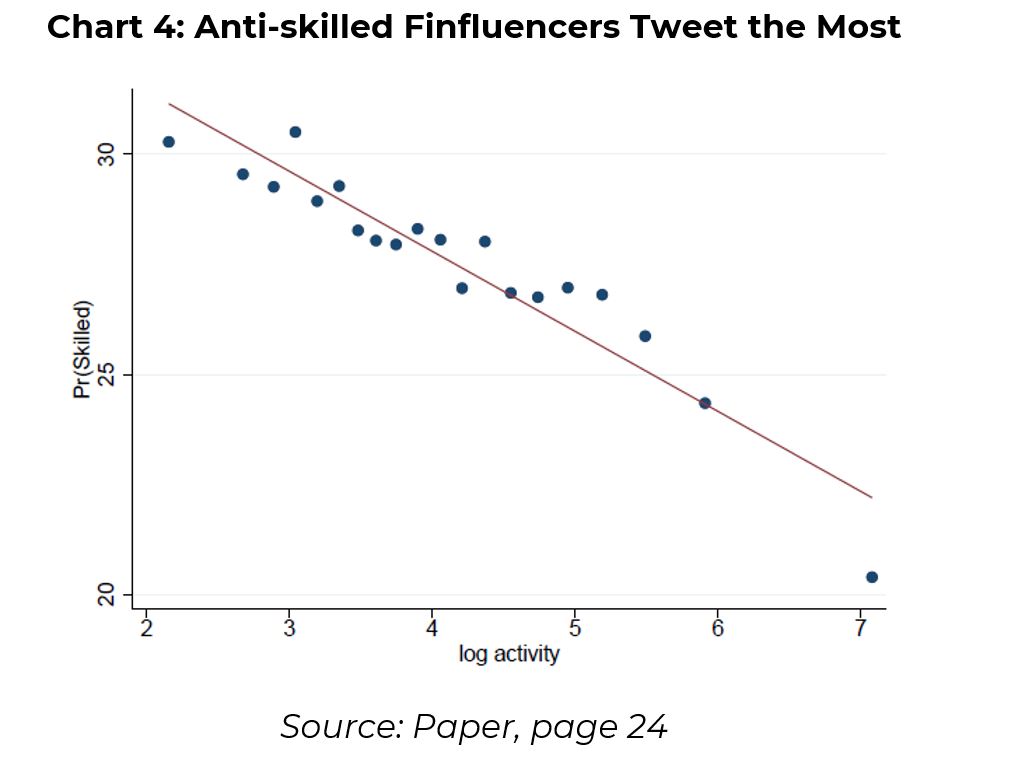
The imposter filter does not stop there. Anti-skilled finfluencers are more likely to ride return momentum, i.e., make positive tweets after positive returns and negative tweets after negative returns. They are also more inclined to jump onto social momentum, by tweeting positively about the latest hottest stocks and negatively on expected losers.
In contrast, skilled finfluencers are more likely to be contrarian. They do not chase returns and do not herd on other users’ tweets. They are also more likely to tweet negatively about overpriced stocks (i.e., ones with a high Markit short-selling index), implying they have a more nuanced understanding of markets.
Overall, following the advice by anti-skilled finfluencers creates overly optimistic beliefs most of the time, since their tweets tend to be bullish about most stocks. Moreover, the social media sentiment surrounding these finfluencers is highly persistent and induces long swings in followers’ belief bias.
According to the authors, the results show that ‘the message is more important that the messenger’ on social media. Following a buy-and-hold strategy against the advice of anti-skilled finfluencers leads to 1.2% monthly abnormal returns!
Bottom Line
Social media users can and should use tweeting behaviour to identify skilled finfluencers. However, a striking feature of the data is that the experts have fewer followers than, what the authors class as, anti-skilled finfluencers.
That result is fascinating. Social media brings together large groups of individuals. Such an agglomeration is usually thought to improve the speed of learning and information diffusion. However, it appears for retail traders, that is not the case.
Consistent with theories of homophily, the paper’s results imply that social media users tend to match with finfluencers that are also no experts. Perversely, this means a reduction in the pace of learning and knowledge diffusion on social media platforms!
Perhaps it is best to avoid social media platforms to inform your trading all together…
Sam van de Schootbrugge is a Macro Research Analyst at Macro Hive, currently completing his PhD in Economics. He has a master’s degree in economic research from the University of Cambridge and has worked in research roles for over 3 years in both the public and private sector. His research expertise is in international finance, macroeconomics and fiscal policy
Photo Credit: depositphotos.com