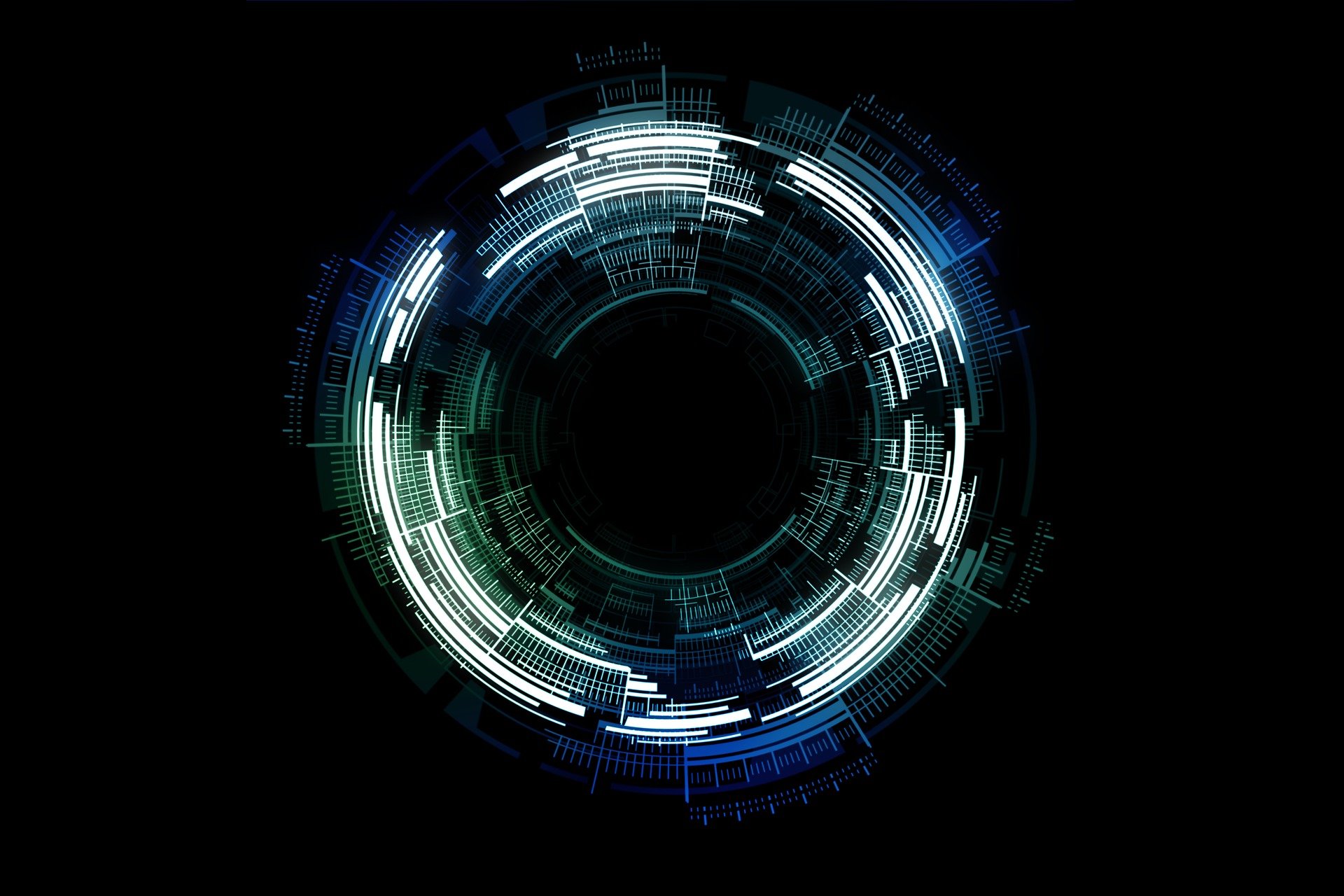
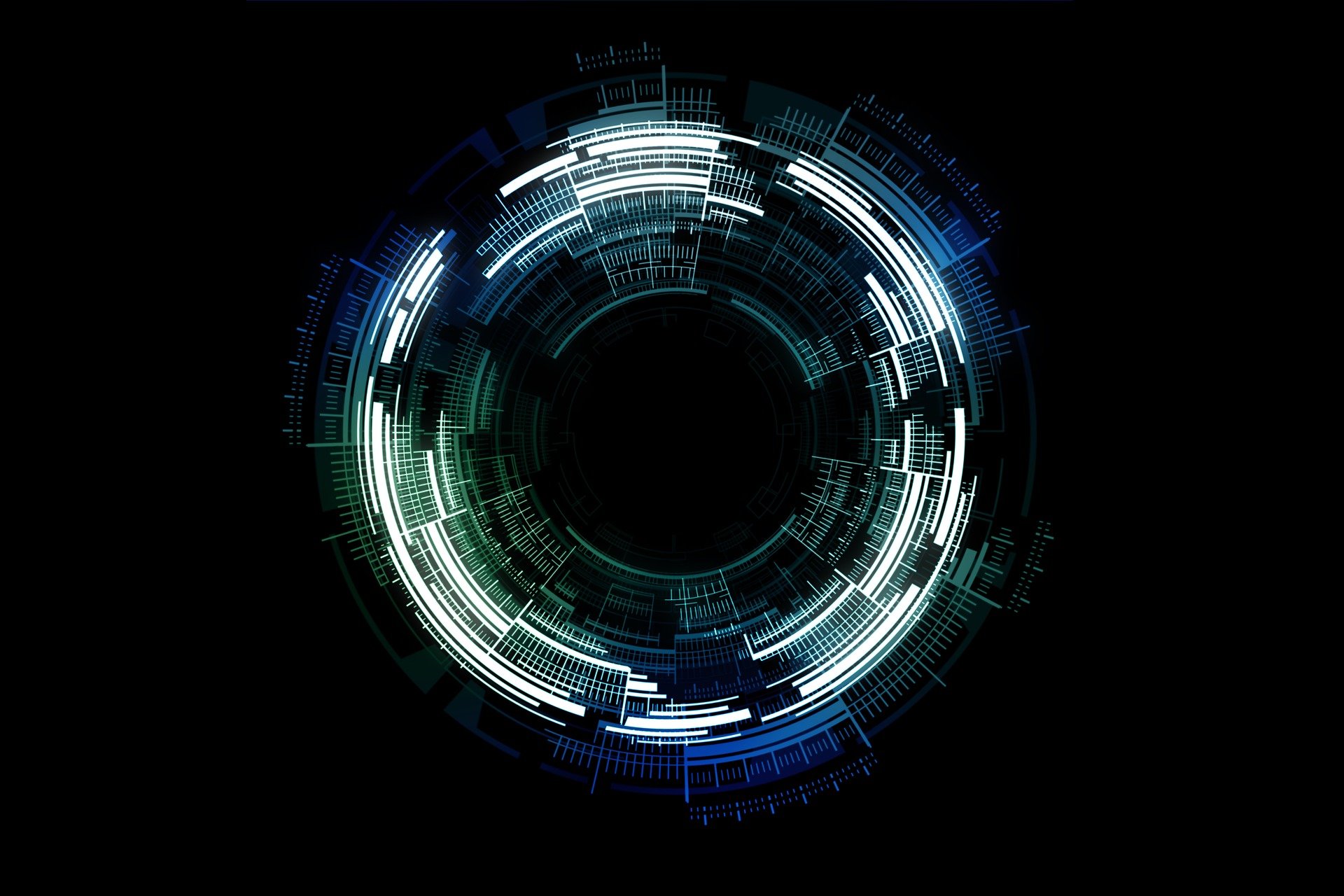
Summary
- A new IMF paper explores the opportunities and risks of artificial intelligence and machine learning in finance.
- It explains how adoption has differed across the financial sector, and that central banks are adopting ML methods slowly.
- The paper identifies six key risks of adopting AI/ML systems: cybersecurity, embedded biases, interpretability, data privacy, robustness and financial stability.
Introduction
Artificial intelligence (AI) and machine learning (ML) are transforming the financial sector. A 2020 World Economic Forum survey showed over 75% of financial institutions anticipate that AI will be of high or very high overall importance to their business within two years. This percentage has increased since the pandemic. McKinsey estimates that AI could potentially deliver up to $1tn of additional value each year to the banking sector.
Yet alongside greater opportunities – cost savings, analytics, fraud management and more – unique risks could create financial instability. A new IMF paper surveys the opportunities and risks associated with AI/ML systems. It also provides insight into where policymakers may turn when dealing with the consequences of new digital technology. It finds:
- AI adoption in central banks has been far slower than elsewhere in the financial sector, such as investment management.
- The widespread adoption of AI/ML systems in the financial sector has six key risks, including cybersecurity, biases and financial stability.
- Cooperation and knowledge sharing at the regional and international level are increasingly important to avoid technological advances outpacing existing regulation.
AI in Forecasting: ML vs Econometrics
We begin, as the paper does, with forecasting. To understand why AI/ML are better forecasting systems, we should note the difference with econometrics. I build on the arguments provided by Prof. Susan Athey.
The focus in econometrics is on obtaining unbiased estimates of a causal relationship between dependent and independent variables. To do so, researchers create structural models based on economic theory, with many assumptions given to us by an ‘invisible hand’. These models’ performance is then evaluated against a handful of other regressions and in-sample statistics, like the mean squared error or standard errors.
Most regressions are notoriously poor at modelling non-linear relationships. Because they seek causal relationships, they prioritise variations in the data away from some trend, rather than fitting the best possible model. And so, in situations with many variables or correlations in the data, it becomes nearly impossible for a linear regression to spot all the causal relationships and then also extrapolate into the future.
In ML, the focus is on fitting a model that can produce the most accurate out-of-sample predictions. Researchers do this by repeatedly estimating a model on part of the data (learning) and then testing it on another part. The models are data-driven so tease out hard-to-detect relationships. They can also be tuned with additional assumptions, and, to ensure models do not become too complex, they are cross-validated.
And so, AI/ML systems make lower forecast errors than econometrics models. One challenge, however, is that ML models lack causal reasoning, which econometrics prioritises above all else. Therefore, many researchers combine the two strands and use AI/ML as inputs into traditional models. One can, for example, use ML to include unstructured datasets and non-traditional data to improve forecasts.
AI in Finance
In the financial sector, advances in AI/ML in recent years have impacted the investment management industry most (Box 2). They are introducing new market participants (e.g., product customisation), improved client interfaces (e.g., chatbots), better analytics and decision-making methods, and cost reduction through automated processes.
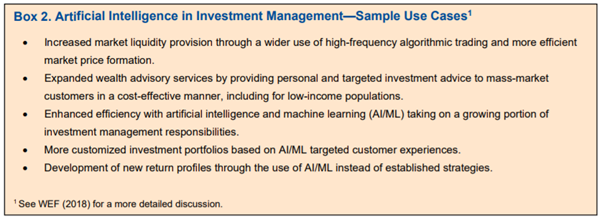
Compared with the investment management industry, the penetration of AI/ML in banking has been slower due to confidentiality and the proprietary nature of banking data. In recent years, penetration has increased, partly due to competition from fintech companies. One area in which AI/ML has expanded is credit underwriting, where ML has been shown to reduce banks’ losses on delinquent customers by up to 25%.
AI and ML are also reshaping regulatory technology (regtech) and supervisory technology (suptech). In regtech, ML methods are the top technology under consideration among firms in the space (Chart 1). In suptech, use cases are mainly concentrated in misconduct analysis, reporting, and data management. However, many supervisory authorities are looking to include AI/ML in microprudential supervision.
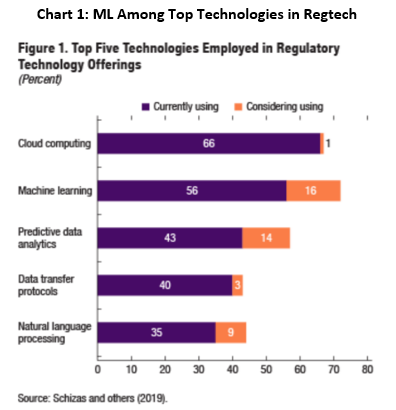
Lastly, according to the authors, central banks have been slow to embrace AI/ML. This is despite the improvements it offers to their operations, systemic risk monitoring, and tuning of monetary and macroprudential policies. Despite the technology already existing, the authors believe the culture, politics and legal implications are restraining central banks. Box 6 from the paper shows how some central banks are using AI.
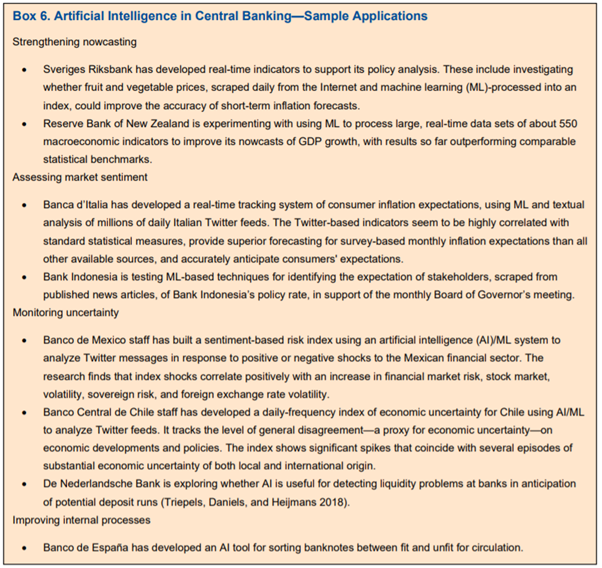
Risks of AI and ML
1) Embedded Biases
The first risk is ‘embedded biases’, where computer systems discriminate against certain individuals or groups of individuals. This happens if the underlying data on which AI systems are trained (i) contains prejudices, and/or (ii) is under-representative of the true population. It may also exist if the researcher includes or excludes various parameters from the ML model.
Data biases could result in greater financial exclusion and feed distrust for the technology, especially among the most vulnerable. On the other hand, ML models also reduce biases. For example, they eliminate irrational decisions. Arguably, they are also easier to scrutinise than individuals who base decisions on subjective data. In recent years, several frameworks have been launched to avoid embedded biases in ML, like the Ethics Guidelines for Trustworthy AI in the EU.
2) Unboxing Black Boxes
The characteristics of AI/ML can make the appropriateness of ML decisions hard to decompose. That, for many, undermines their capabilities. Surely, knowing why an individual was granted or refused credit is important. There are still very few industry-wide regulations on this front; however, some models can aid interpretation, such as linear models and decision trees, but would not be used for prediction.
According to the authors, research is increasingly focused on developing techniques that use ML models or their outcomes as training input to achieve explainability. This can be done by repeatedly disturbing the ML inputs or variables and comparing them with the ML outcome to ascertain the decision-making process. However, there is no assurance this can reasonably explain the results of a complex ML model.
3) Cybersecurity
The greater the AI adoption, the greater the scope for cyber threats. This could involve manipulating the data to create biases. These attacks, also known as ‘data poisoning’, cause the AI to incorrectly learn to classify or recognise information. Alternatively, input attacks allow attackers to introduce perturbations to data inputs and mislead AI systems during operations. This could, for example, allow hackers to evade detection.
Lastly, model extraction or model inversion attacks attempt to recover training input and data, or the model itself. Such attacks may be performed as black-box attacks, whereby attackers have merely read-only access to the model, possibly through business application programming interfaces. Current privacy protection laws are not designed to deal with the methods of model inversion attacks, raising privacy and copyright concerns.
According to the IMF, cyber security attacks are becoming a concern for financial sector regulators. Corrupted systems could undermine the financial sector’s capacity to accurately assess, price, and manage risks, which could allow unobserved systemic risks to build up.
4) Data Privacy
It is well known data attributable to individuals is anonymised. However, AI/ML models can unmask anonymised data through inferences. Similarly, AI/ML may remember information about individuals in the training set after the data is used, or AI/ML’s outcomes may leak sensitive data directly or by inference. Tools are being developed to address these issues and strengthen AI/ML models’ robustness in safeguarding sensitive data, but, according to the authors, more work is needed.
5) Robustness
So far, financial sector AI/ML models have performed well in a stable data environment. But they have been known to accentuate risks in periods of rapid structural shifts. Many jurisdictions lack adequate oversight of AI/ML service providers. New governance frameworks for the development of AI/ML systems are needed to strengthen prudential oversight and avoid unintended consequences.
6) Impact on Financial Stability
Knowing whether AI will improve financial stability is hard because it could cause new sources and transmission channels of systematic risk. For example, AI/ML service providers will likely become more important participants in financial markets, leading to greater vulnerability to single points of failure. Also, regulatory gaps could affect financial stability if tech progress outpaces existing regulations.
AI/ML could also increase the procyclicality of financial conditions, and a lack of interpretability could obscure issues before they arise. In tail risk events, algorithms could amplify shocks, and these could spread throughout the financial system faster. According to the IMF, lots still needs to be done to implement regulations to mitigate these risks.
Bottom Line
The widespread deployment of AI/ML systems in the financial sector will be transformational. These systems may bring increased efficiencies; better assessment, management, and pricing of risks; improved regulatory compliance; and new tools for prudential surveillance and enforcement. However, they will also bring new and unique risks arising from the opacity of their decisions, susceptibility to manipulation, robustness issues, and privacy concerns. The IMF paper summarised here provides a clear high-level overview of these risks.
Citation
Boukherouaa, E.B et al (2021) ‘Powering the Digital Economy: Opportunities and Risks of Artificial Intelligence in Finance‘, International Monetary Fund
Sam van de Schootbrugge is a Macro Research Analyst at Macro Hive, currently completing his PhD in international finance. He has a master’s degree in economic research from the University of Cambridge and has worked in research roles for over 3 years in both the public and private sector.